Enhancing human capital management through GPT-driven questionnaire generation

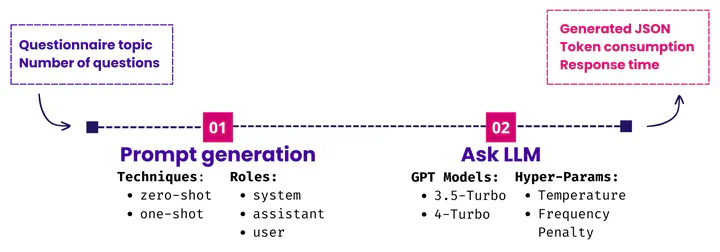
Survey questionnaires capture employee insights and guide strategic decision-making in Human Capital Management. This study explores the application of the GPT-3.5-Turbo and GPT-4-Turbo models for the automated generation of HR-related questionnaires, addressing a significant gap in the literature.
We developed a novel dataset of HR survey questions and evaluated the modelsβ performance using different task configurations, including zero-shot and one-shot prompting with various hyperparameter settings. The generated questionnaires were assessed for instruction alignment, syntactic and lexical diversity, semantic similarity to human-authored questions, and topic diversity, or serendipity. In collaboration with Talentia Software, we additionally examined the indistinguishability of AI-generated content from human-created counterparts.
Results indicate that both models produce questionnaires with high serendipity and intra-questionnaire diversity. However, the indistinguishability test revealed that human evaluators could still distinguish AI-generated content, particularly noting differences in language style and answer variability. These findings underscore the potential of GPT-driven tools in automating questionnaire generation while highlighting the need for further refinement to achieve more human-like outputs.